The decisions doctors make are a question of life and death. They relate to everyone. To make correct decisions, medical care professionals need to process and analyze enormous volumes of data, with every detail taken into consideration. Unfortunately, human factors and human errors are always at play. People, even the most experienced and highly qualified ones, make mistakes. In this aspect, predictive modeling comes to the rescue. Indeed, predictive analytics in healthcare is already helping medical professionals mitigate risks. Available statistics corroborate this statement. As the experts employed by Acumen Research and Consulting stress, back in 2021, the global predictive analytics market in healthcare amounted to $9.5 million. Around 2030, it is projected to hit $87.5 billion. As for the current rates of use of predictive analytics in healthcare, per Statista, they are also impressive:
- Global average — 56%;
- Singapore — 92%;
- China — 79%;
- US — 66%;
- India — 59%;
- Australia — 55%.
As you can see, predictive health analytics solutions are already in high demand. Below, we will explore the most important aspects of this growth. Our extensive and multi-faceted experience in this domain undoubtedly makes us a reliable source of expertise.
What is predictive analytics in healthcare?
It is the process of analyzing both present-day data and historical records and trying to identify, on this basis, new opportunities to make more workable clinical and managerial decisions, foresee trends, fight pandemics, and improve overall health. As you might have guessed, the sources of Big Data required for that may include EHRs, administrative papers, insurance archives, collections of medical images, etc. Whatever combination of sources you may be using, the ultimate goals remain the same. Namely, to build a predictive model relying on empirical information. Hence, the typical sequence the solutions perform is:
- Mine and structure data;
- Apply both existing statistical models and ML tools to that data;
- Specify and describe the resulting patterns to ensure the best possible outcomes.
Benefits of predictive analytics in healthcare
The key mission of medical professionals is to save people’s lives and cure them when they get ill. Their second priority is to help people stay healthy so that treatment and interventions aren’t needed. Finally, healthcare is expected to stay affordable, cost-effective, and transparent as a system. Predictive analytics in healthcare helps us achieve these requirements by:
- Identifying the cohorts of patients and potential patients under the greatest risks. While it is not impressive to deduce that chain smokers and heavy binge drinkers are the most obvious at-risk groups, healthcare AI software can do much more. For instance, it is already possible to identify groups of diabetic patients who are most likely to be hospitalized on the basis of their age, gender, lifestyle, co-existing health conditions, adherence to medical routines, exercise habits, etc.
- Mitigating public health risks by recognizing outbreaks and other health emergencies in advance.
- Streamlining administrative tasks, speeding up medical responses and timely hospital discharges, as well as enhancing insurance procedures to allocate money more effectively.
- Lowering the costs spent on regular appointments, no shows, and inevitable hospital readmissions. By leveraging the ML and AI-fueled capabilities, we can not only forecast health dynamics, but also behavior patterns of various groups to prepare for potential problems.
- Streamlining administrative tasks, speeding up medical responses and timely hospital discharges, as well as enhancing insurance procedures to cut costs and allocate money and effort more effectively.
- Boosting patient engagement and adherence rates by prescribing efficacious regimes.
In fact, predictive health analytics can be helpful and effective across an entire range of no-so-obvious spheres, such as suicide and overdose prevention. Let’s examine several of the most promising cases of use of predictive analytics in healthcare.
Predictive analytics use cases in real life
To begin with, no matter how complex certain models, both human- or AI-generated ones, may seem to be, their key foundations are quite understandable:
- Logistics regression aimed at predicting a data value on the basis of earlier observations and data sets;
- Decision trees, i.e. graphs applying the branching method to indicate likely consequences of any decision made;
- Time-series analysis, that is to say, time-based projections of data points at certain moments.
With these techniques at our disposal predictive health analytics is capable of:
- Calculating risk scores when it comes to chronic conditions, which are used by prevention clinics, insurance companies, and government-level decision-makers;
- Preventing cases of patient self-harm, as mental health professionals are informed of early indicators and predictors of instability;
- Foreseeing rapid health deterioration cases, which means the professionals are able to react faster to changing vitals and take action before dangerous symptoms become obvious;
- Rapid clinical decision-making enabling doctors to rely on external data-driven automated support when somebody’s life is at stake;
- Fighting fraud, in terms of both patients’ false reports caused by mental conditions, desire to quit a job, get unearned benefits, or swindle the insurance company;
- Designing optimized treatment routines and medical care procedures to attain the best possible results based on available data.
Whatever your particular type of solutions may be — there are many more than those mentioned above — their key dimensions would be as follows. This is an approximate list of predictive analytics in healthcare examples:
- Diagnosing conditions and forecasting their developments;
- Increasing the quality of medical care while cutting costs;
- Generating treatment recommendations and providing support for decision-making;
- Mitigating treatment risks and side-effects while ensuring proper remote monitoring.
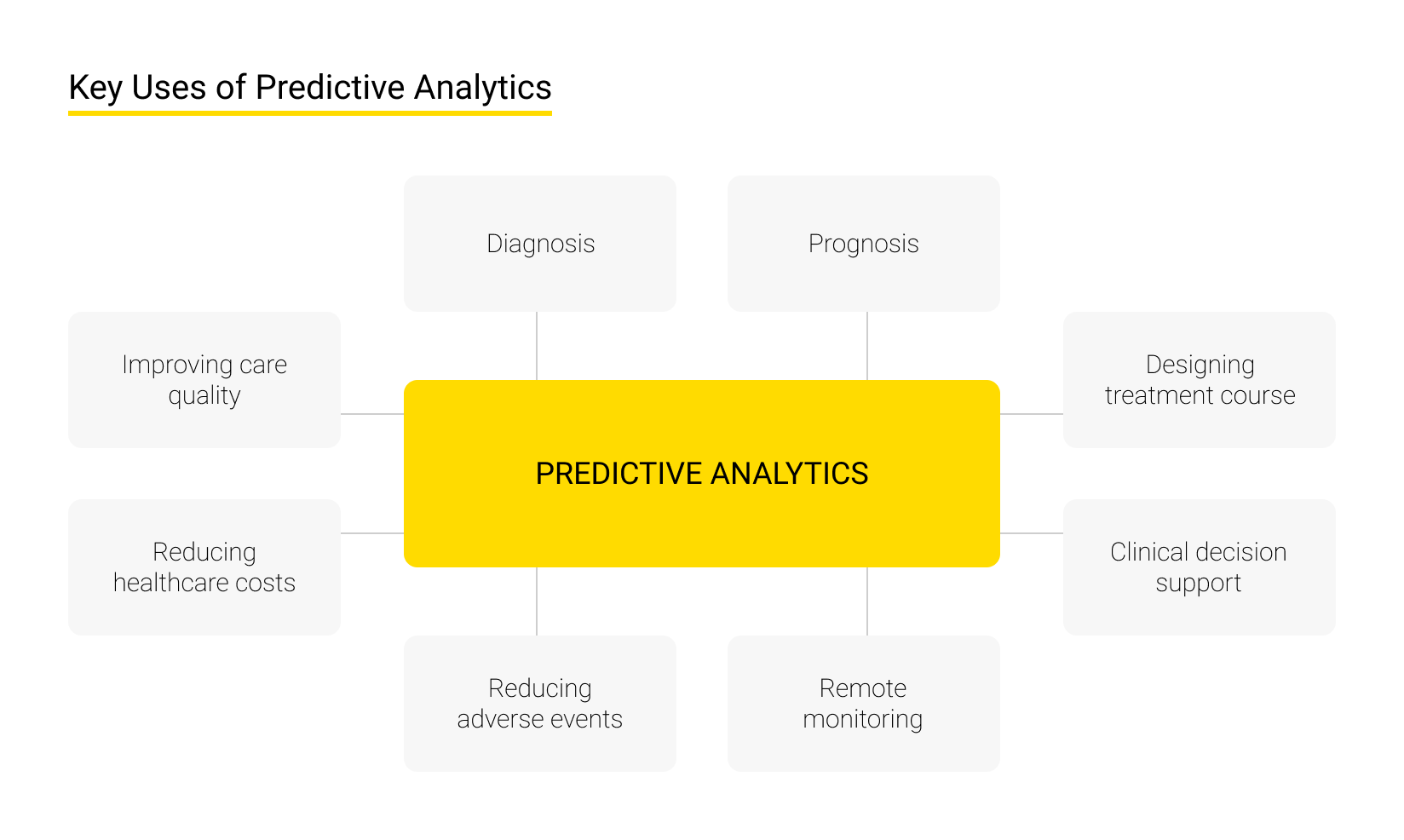
Predictive analytics challenges in the healthcare domain
All that optimism notwithstanding, predictive analytics in healthcare does face a range of challenges. The problem is that, when it comes to healthcare, human lives are at stake. Correspondingly, the price paid for a mistake is universally high, whatever the potential benefits of predictive analytics Hence, precaution is always needed in such fields as:
- Proper data collection, cleaning, and storage. That is to say, data has to be seamlessly transmitted via effective wearables and other IoT devices, correctly arranged, and stored in a reliable fashion, either on the in-house server or in the cloud;
- Medical records. These are, by definition, sensitive information protected by numerous laws and regulations. Hence, any software engineer, any QA expert, and any database manager has to be a certified professional;
- Adoption. The issue is that not every nurse or doctor is tech-savvy. That is doubly true for patients, especially, when it comes to the elderly or mentally-challenged people. Hence, the products — especially when it comes to wearables — have to be convenient-to-use, easy-to-read, and completely accessible.
Conclusion
Technologies delivered by reliable custom software development vendors are groundbreaking. Andersen’s expert-level team of developers, designers, and QA engineers is ever-ready to help you with implementing predictive health analytics initiatives, whether they are AI-powered healthcare products in general or AI-based imaging solutions in particular.
Reach out to Andersen, schedule a free consultation, outline your requirements, and we will start delivering the best possible results ASAP. Our experience, customer reviews, and professional advisory board made of true experts are the best guarantee of your success in predictive healthcare.
Our expert is ready to help you with complex processes. Schedule a free consultation.
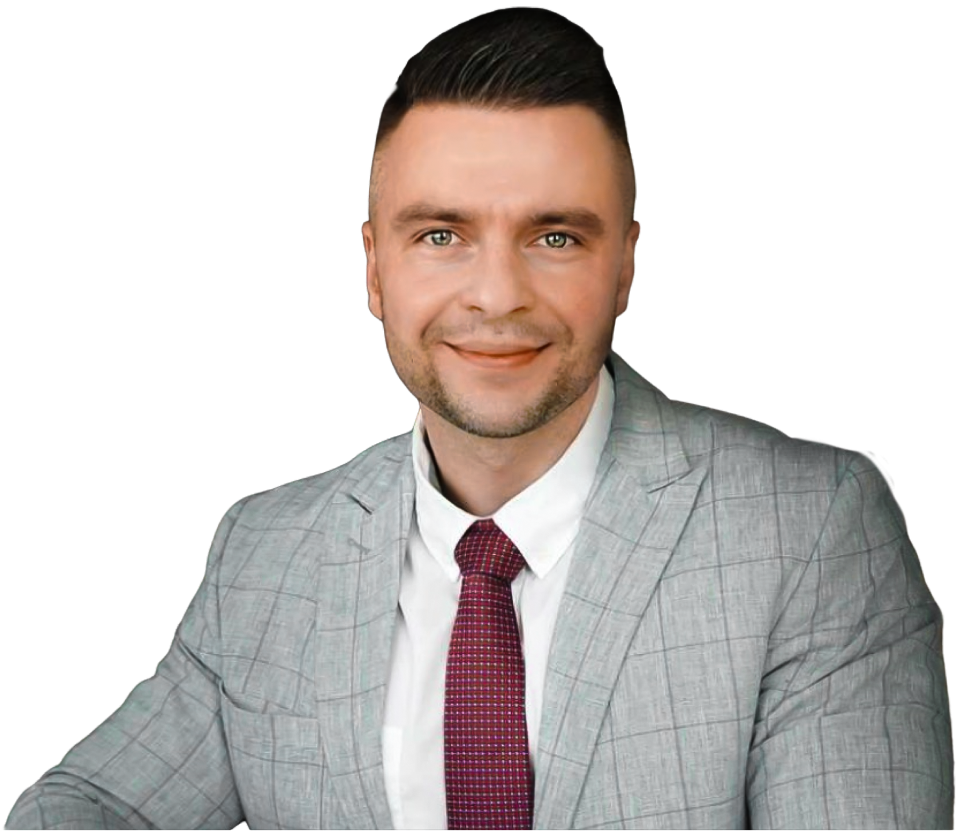
Book a free IT consultation
What happens next?
An expert contacts you after having analyzed your requirements;
If needed, we sign an NDA to ensure the highest privacy level;
We submit a comprehensive project proposal with estimates, timelines, CVs, etc.
Customers who trust us